Main content
Top content
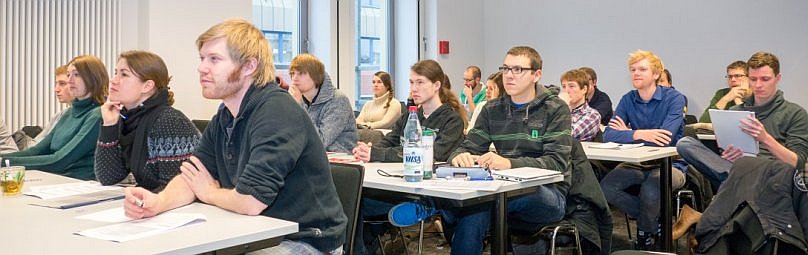
Research Seminar Systems Science
Winter semester 2021/22
Virtual seminar in BigBlueButton (https://webconf.uni-osnabrueck.de/b/fra-h1b-axo-fe5). Entry code available upon request.
Timetable
Date | Time | Presenter | Title | Notes | |
---|---|---|---|---|---|
25.10.2021 | 2:15pm | Sonja Türpitz, Friedrich-Schiller-Universität Jena | Mutualism, poisoning, and modeling the lung microbiome | ||
16.11.2021 | 3:00pm | Stefan Kittelmann | Ein georeferenzierter Modellansatz zur Abschätzung des erosiven Mikroplastikeintrags aus Klärschlamm im Einzugsgebiet der bayerischen Donau (Bachelorarbeit) | 66/E01 | |
06.12.2021 | 2:15pm | Dr. Samuel Fischer, Osnabrück University and Helmholtz Centre for Environmental Research | KDE-likelihood: a tool for fitting agent-based models to equilibrium data | ||
17.01.2022 | 2:15pm | Friedemann Liebaug | Part I | ||
31.01.2022 | 2:15pm | Friedemann Liebaug | Part II | ||
21.02.2022 | 2:15pm | Paul Weigel | Holistic evaluation of digital applications in the energy sector: Evaluation framework development and application to the use case smart meter roll-out | ||
Abstracts of selected talks
Samuel Fischer, December 6, 2021
KDE-likelihood: a tool for fitting agent-based models to equilibrium data
Fitting stochastic agent-based models (ABMs) to observational data can be challenging, because the complexity of ABMs typically prohibits direct application of classical statistical tools such as the likelihood. Hence, modellers often examine the parameter space by applying sampling-based methods such as approximate Bayesian computation (ABC), or they reduce stochasticity by aggregating results over many simulation runs. However, if the available data represent a system in equilibrium state, sampling may be computationally costly, since long simulation runs may be required to reach this state. This makes methods such as ABC inapplicable. At the same time, aggregating results may lead to information loss that could result in identifiability issues corrupting the reliability of the parameter estimates. In this talk, we suggest the kernel-density-estimate-based (KDE-) likelihood as a tool circumventing these issues. The KDE-likelihood allows modellers to exploit the favourable statistical properties of the likelihood function without deriving it in closed form. We introduce our approach using a simple ‘toy’ model and showcase the method's advantages in real applications by fitting the forest model Formind to forest inventory data from Changbaishan, China.